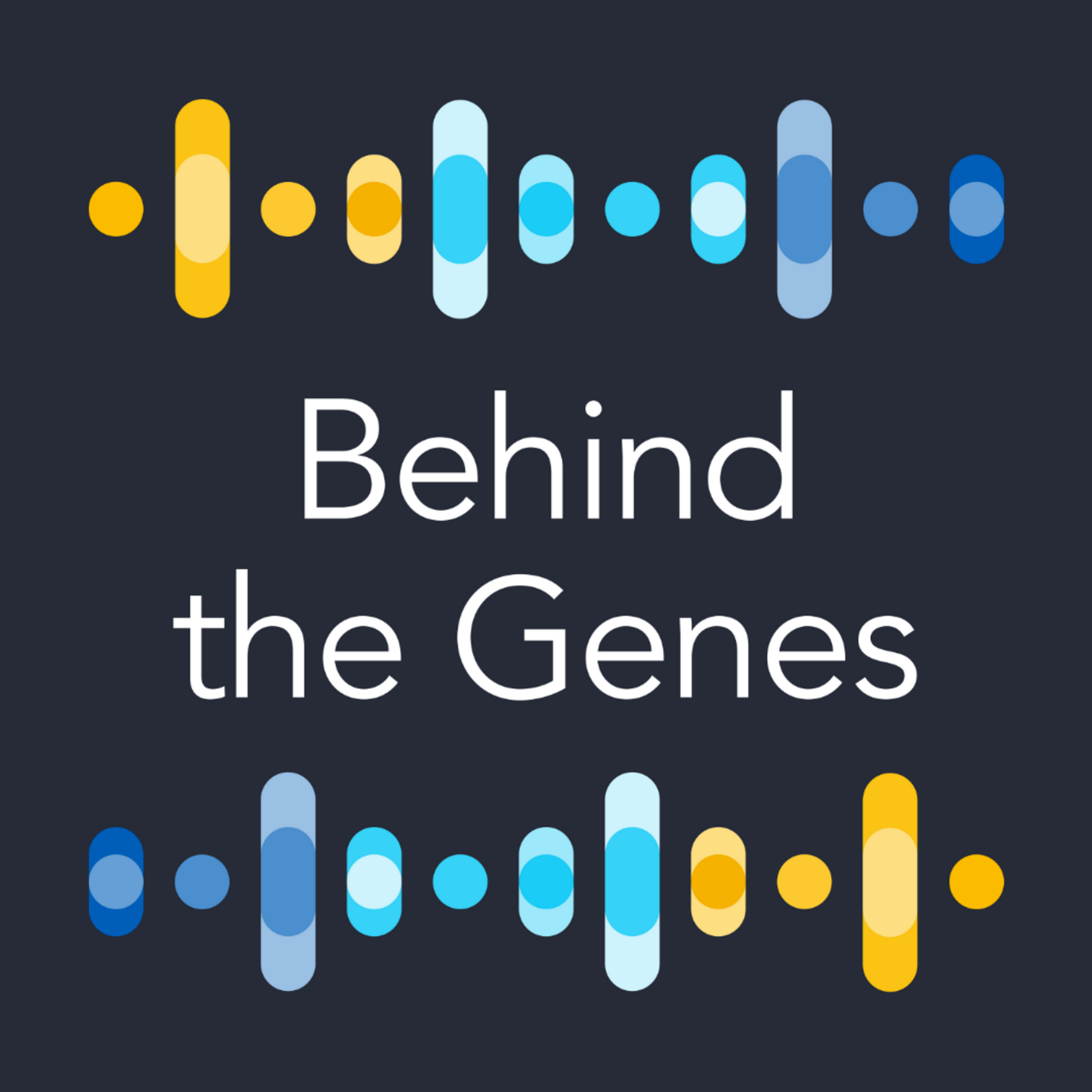
We are Genomics England and our vision is to create a world where everyone benefits from genomic healthcare. Introducing our refreshed podcast identity: Behind the Genes, previously known as The G Word. Join us every fortnight, where we cover everything from the latest in cutting-edge research to real-life stories from those affected by rare conditions and cancer. With thoughtful conversations, we take you behind the science. You can also tune in to our Genomics 101 explainer series which breaks down complex terms in under 10 minutes.
Episodes
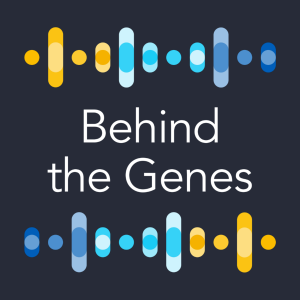
Wednesday Jan 31, 2024
Wednesday Jan 31, 2024
On this episode, we delve into the promising advances that artificial intelligence (AI) brings to the world of genomics, exploring its potential to revolutionise patient care. Our guests discuss public perspectives on AI in genomics and address the ethical complexities that arise in this rapidly evolving field. Gain valuable insights into the future landscape of genomics and AI, as our experts discuss what to expect on the horizon.
Our host Dr Natalie Banner, Director of Ethics at Genomics England, is joined by Ismael Kherroubi García, member of the Participant Panel and Ethics Advisory Committee at Genomics England, and Francisco Azuaje, Director of Bioinformatics at Genomics England.
“So, AI is already driving the development of personalised medicine for both research and healthcare purposes. [...] In the context of healthcare, we are talking about AI tools that can support the prioritisation, the ranking of genomic variants. To allow clinicians to make more accurate and faster diagnosis.”
You can download the transcript or read it below.
Natalie Banner: Hello, and welcome to the G Word. In the past few years, artificial intelligence, or AI as a shorthand, has taken centre stage in the headlines. Sometimes for really exciting, positive reasons about the potential to drive improvements for society, and sometimes because of its potential risks and harms. These discussions and stories can sometimes seem like they're straight out of science fiction. There are a lot of questions, excitement, concerns about the societal impact of AI, so not just looking at individual patients, but that broader what does this mean for society?
Ismael Kherroubi García: My somewhat hot take is that AI only accelerates societal impacts that genomics research and healthcare can have. So the impacts, of course, will be diverse and complex and quite widespread, especially given the quite nuance and sometimes difficult to understand areas of genomics and artificial intelligence. But the key takeaway from what I want to say is that it only accelerates the impacts of genomics and healthcare. So if we take genomics research to promote human flourishing, ideally, artificial intelligence will also only help further human flourishing. Conversely, applying artificial intelligence tools to genomics research can help perpetuate certain stereotypes and related harms.
Natalie Banner: My name is Natalie Banner, and I'm the Director of Ethics at Genomics England. On today's episode I'm joined by Ismael Kherroubi García, member of the Participant Panel, and Ethics Advisory Committee at Genomics England. And Francisco Azuaje, Director of Bioinformatics at Genomics England. In today's episode we aim to cut through the hype and hyperbole and explore the real possibilities for AI within the domain of genomics and healthcare. We'll look at how AI tools and techniques have been used to date, and what the future holds, considering both the benefits and challenges faced in the genomics ecosystem. If you enjoy today's episode, we'd love your support. Please like, share, and rate us on wherever you listen to your podcasts.
AI is in the news an awful lot, and not always for good reasons. There are many big and small tech companies that are exploring the use of AI in all walks of life, from finance to retail, to healthcare. And it's not always clear what AI means in these contexts, where it actually has the potential to really help people, drive improvements to healthcare and society, for example. But there are some exciting stories, so recently, Genomics England undertook a collaboration with DeepMind on their AlphaMissense tool, and that sought to classify the effects of 71 million missense mutations in the human genome. So it could process data at a scale and a speed far faster than any human has ever been able to before. So there's an awful lot of exciting work going on in AI, but we should emphasise that although some of this technology is really cutting edge, a lot of the techniques that are being used and talked about in AI, have actually been around for quite a long time. So Francisco, if I could start with you, can you help us understand what artificial intelligence, AI really is in the context of genomics? And maybe explain to us the difference between AI and machine learning, and how they relate to one another?
Francisco Azuaje: Sure, Natalie. AI involves the creation of computer systems capable of performing tasks that typically require human intelligence, such as understanding natural language, recognising patterns in big data sets, and making decisions. Now, machine learning is the most successful technology within the field of AI, but machine learning focuses on the use of algorithms that allow computers to learn from the data and make predictions about the data without the need for explicit programming for each task or application.
Natalie Banner: Ismael, perhaps I can turn to you. What do you see as the primary motivations or reasons for incorporating AI into genomics research and, indeed, into healthcare?
Ismael Kherroubi García: So I think it's already been mentioned and focusing on genomics research because challenges, the enormous amounts of data that are required to shift through and analyse and get insights from. So one number that's worth just mentioning is that the human genome is made up of 3.2 billion base pairs, As with the Ts and the Gs with the Cs in our DNA. And one way to put that 3.2 billion, with a B, in terms we might understand, is to say that to list all of those letters we would have to be typing 60 words a minute, eight hours a day for about 50 years, that's how enormous just one human's genome is. And I kept looking for other ways of depicting just how enormous this data set is, and it turns out if you uncoil those strands that we usually see depicted when talking about DNA, if we uncoil them for one person, we would have a string about 67 billion, again with a B, miles long for each person, that's roughly 150,000 round trips to the moon. So, again, this is just one person, those numbers are enormous.
It's also worth considering the role technology has had to play in enabling genomic research. So if we look back at maybe a very significant catalyst for genomics research, the human genome project which started in 1990, they took 13 years to sequence one human genome. Now, what we're talking about is estimating that by 2025 genomic-related data will be up to 40 exabytes of data. Now I didn't even know what an exabyte even was before this podcast, so I did look it up, that's about a billion gigabytes, I definitely don't know how to even begin to imagine what 40 exabytes even means. Bringing it a bit closer to home, I try to figure out how many copies of Doctor Who we would need to make 40 exabytes of data, I found that Doctor Who is roughly 850 gigabytes, I found this on Reddit, very scientific. And the number is then 40 exabytes over 850 gigabytes, that's roughly 47 million copies of all the decades of Doctor Who media is what's necessary to reach the amount of data we expect from genomic research within a couple of years. So we need a technology capable of analysing the equivalent of 47 million copies of the entirety of Doctor Who, and currently, as you've both mentioned, AI provides the best way we have to do this.
Natalie Banner: Wow! So we are talking absolutely vast amounts of data. And I do love the analogies there, it's very helpful to actually sort of bring it home and make it real. So we're talking vast amounts of data and currently it feels as thought AI may be the best way to try to analyse and explore that scale of data. So given that's what we're talking about in genomics, in what ways is AI currently being applied in the field of healthcare and genomics? Francisco, I'm wondering, can you give us any examples of how Genomics England is integrating AI models and tools into its research efforts? And I know particularly we have a programme of work exploring multimodal data, can you tell us a little bit about that?
Francisco Azuaje: Absolutely. But first of all, just to give you an overview of the type of applications in research and healthcare, right now AI offers opportunities to develop tools that are needed to support interpretation of genomic variants, and the relationship between those variants and medical conditions, drug responses. AI is also a powerful approach to supporting the detection of diseases and some subtypes of these conditions, and matching those conditions to treatments, using different types of data, in the clinic this is happening already in the clinic, and examples of data include medical images, clinical report, electronic health records. So AI is already driving the development of personalised medicine for both research and healthcare purposes.
Now, at Genomics England we are investigating the use of AI to support a number of tasks with potential impact in both research and healthcare. In the context of healthcare, we are talking about AI tools that can support the prioritisation, the ranking of genomic variants to allow clinicians to make more accurate and faster diagnosis. You mentioned the multimodal programme at Genomics England, as part of our mission to enabling research, we are developing tools and applications to help researchers extract information from different modalities of data or data types. In this context, AI plays a crucial role to deal not only with the size and the volumes of this data, but also to allow the meaningful extraction of useful information with clinical value based on the combination of different data sets. And that's a complex challenge, that only AI can approach. Here, we're talking about large, diverse, and complex data sets coming from different types of clinical imaging modalities. We are talking of course about genomic data, clinical reports, and in general any information that is included in the patient's medical health record.
Natalie Banner: Thanks, Francisco. And can you talk a little more about the specific tools or projects you're working on at the moment in multimodal?
Francisco Azuaje: Absolutely. So, in the case of multimodality, we are talking about applications that aim to improve the way we connect any of these data sources, including imaging and genomics, with clinical outcomes. For example, how to improve the way we predict not only a diagnostic type, but also how that information can be correlated with the potential response of a patient to a particular therapy. Or a prediction of the potential evolution of that patient within a particular subtype of condition or phenotype. To do this we rely on a type of a machine learning technique called deep learning, just very briefly, deep learning models are again a branch of AI, so within machine learning, these models are very powerful tools that apply deep neural networks. These networks consist of multiple layers of mathematical transformations that are applied to the data. And these transformations allow them the automatic discovery of complex patterns in this data, including all these modalities that I mentioned before. So this is a key approach that we need to extract useful features with diagnostic or prognostic value from these different modalities of clinical information.
Natalie Banner: So there's obviously a really clear focus there on the benefits to patient, the patient outcomes, really trying to ensure that we can create personalised medicine as far as possible. That every patient can have an outcome that's kind of very much about their own particular circumstances and condition. So not just looking at individual patients, but broader, what does this mean for society? Ismael, I wonder if you can tell us a little bit, your thoughts on the questions about societal impacts with the increasing use of AI, particularly in genomics and healthcare more widely?
Ismael Kherroubi García: Yeah. So my somewhat hot take is that AI only accelerates societal impacts that genomics research and healthcare can have. So the impacts, of course, will be diverse and complex and quite widespread, especially given the quite nuanced and sometimes difficult to understand areas of genomics and artificial intelligence. But the key takeaway from what I want to say is that, it only accelerates the impacts of genomics and healthcare. So if we take genomics research to promote human flourishing, ideally artificial intelligence will also only help further human flourishing. Conversely, applying artificial intelligence tools to genomics research can help perpetuate certain stereotypes and related harms. Genomics England has diverse data initiative and they show that Europeans represent 78% of people in genome-wide association studies.
The challenge here is that, if we train artificial intelligence tools on complex interrelations of mainly the genomes of people with European ancestry, then we are over-sampling people with European ancestry, and the findings will have very limited effectiveness on different populations, both around the UK and diverse populations around the world and within the UK. So whilst artificial intelligence will have societal impacts as a general sort of technology that can be applied to many different fields, in the context of genomics and healthcare, I think that the societal impacts we should really be focusing on relate with genomics and healthcare in particular.
Francisco Azuaje: I agree with Ismael, that the real value of AI is not only in the acceleration of technological progress, but in the impact at different levels of society. Including the way we improve health in an ethical way, and also in the way we support people to develop tools that have an impact in the way we operate as societies and the way we relate to each other. So I totally agree, it's more than just technological acceleration.
Natalie Banner: Absolutely, okay. So we've talked about the potential societal impacts, and I mentioned at the outset that there's a lot of hype and a lot of interesting narratives about AI in the public domain. Things can feel very utopian or dystopian as an awful lot of marketing, but understandably as well a lot of fear coming from the public perspective, especially if you think that most people's understandings of terms like AI have come from science fiction, for example. So, Ismael, what concerns are there from a public perspective? Particularly for, you know, when patients faced with the increasing use of AI and machine learning in genomics and healthcare, and the idea that their care or their treatment could be informed by these tools and technologies. What kind of challenges might arise for patients in the future as these technologies continue to advance? And what are the perceptions like from that public and patient perspective?
Ismael Kherroubi García: I think you got it entirely right. The biggest concern relates with the public perception of AI and that perception in turn is significantly impacted by what we see in mainstream media, be it in the news media, social media in adverts, and so on. And unfortunately, as artificial intelligence is usually depicted as this extremely technical field, the conversation, the narrative is more often than not steered by big tech, so organisations and people with very clear agendas. The example I want to make this case with is this open letter, I think it was in March this year, which was put together by a series of company CEOs, a few researchers as well, and it's ultimately been signed by over 30,000 people. And this open letter called for a six-month pause on what they called 'giant AI experiments'. So this was an open letter and direct response to the launch of ChatGPT, which is an AI-based chatbot launched by Open AI in November 2022.
The open letter suggests that we might, in quotes, that 'we might be developing non-human minds that might eventually out-number and out-smart us. And we're risking loss of control of our civilisation'. These are extremely serious fears, and I would be really afraid if I believed them. So the concern here is that the fears aren't really grounded in reality, but in common fiction or narratives about AI. And very quick way to see that there's a lot of fiction around AI, if you go online, go to your favourite images browser, and look for 'artificial intelligence', you're going to find a lot of images of blue, floating brains, a few versions of the terminator, robot shaking hands with people.
There's one great image of a robot using a laptop – which always makes me laugh. These are not informative depictions of artificial intelligence, let alone genomics. And the risk is ultimately that, if the general public has easy access to unhelpful fictions about AI, then there's a great possibility that genomics research, which is going to remain intricately linked with AI advancements, will be perceived negatively, so genomics services fuelled by AI will not be trusted. And ultimately, given my stance, and I think the shared stance that AI is necessary for genomics, who's going to pay? Well, that will be the patient.
Natalie Banner: So we have quite a battle on our hands, in terms of trying to create space for those informative discussions, as you call them, Ismael, about the realities of what AI can and will be doing in genomics. Francisco, how are we addressing those kind of questions and concerns in our work at Genomics England? What steps do you think we can take at Genomics England to talk more openly about the work that we're doing involving AI to try and create space for those informative discussions that aren't led by the hype or the fears of AI?
Francisco Azuaje: I agree that we have to ensure that we are not distracted from many discussions that emphasise potentially fictional or existential risk of AI. I think there are valid concerns about existential risks that don't represent the AI fictional view of Hollywood, but that really affect the way we operate our societies. For example, existential risk for democracies, if you have monopolies of this technology. If we have less accountability, in terms of governance. If we have electoral systems that do not work. So if that doesn't work it's going to be very hard to benefit from AI within healthcare, so that's something to be considered as well. But I agree that sometimes the discussion is driven by this interest in very long-term potential scenarios. I think the key is to achieve a balance between longer-term and near-term priorities. And in the case of healthcare, there are many challenges and issues that we should be discussing and addressing by now, including challenges regarding the privacy and the respect of rights of our patients and individuals. Concerns about the biases embedded in the data used to build the systems, biases actually embedded in the practices for building the systems. So these are real risks, that in the case of healthcare and research need to be addressed now.
In the case of Genomics England, we are doing a lot of work that is laying the groundwork for safer, ethical uses of AI. So this means, for example, that we will continue doing what we do, inspire and driven by the need to respect our patients' our participants' privacy and rights and voices, so that's essential. In practice, this means that we work closely with our Participant Panel and different committees responsible and accountable for protecting these views and rights. From a machine learning point of view, there are technologies and tools that are quickly emerging that we are using to ensure that our systems are properly designed with ethical considerations in mind.
For example, we ensure that our data sets are of good quality, and good quality means not only the information that we want to use for a particular application, but also means that we identify and quickly mitigate potential biases embedded in the data. It also means that if we share a tool, for example, within our Research Environment, these tools have been properly tested. Not only for reliability but also for potential risks associated with privacy, with biases, etc., before these tools are deployed to a production environment or shared with the wider community. So these are basic steps, but I think they are essential, starting with the protection of our data and also by applying best practice in the way we build and evaluate these models carefully before they are deployed to a wider use.
Ismael Kherroubi García: And that, to me, makes perfect sense, and it's always encouraging to hear the practices around AI and Genomics England. There is one challenge that came to mind that you mentioned, the impact of democratic values, on potentially artificial intelligence informing social media, that informs electoral processes. And there's another very real, tangible issue with artificial intelligence, which is the environmental impact. So what's really interesting, the challenge here is that artificial intelligence tools have significant environmental impacts. You have enormous data centres that need to be submerged in water, maintained, kept cool, and we're developing enormous algorithms, ChatGPT, and so on, that require these huge amounts of data I mentioned earlier on. So there is this really tricky balance between health and the natural environment, which I don't have the capacity to even begin to think about.
So, I sit on the Participant Panel at Genomics England, and the conversation often goes around how Genomics England use our data, how our privacy is preserved. But at the intersection of artificial intelligence in Genomics England, I might have slightly different concerns that don't relate directly with privacy. I usually think about three – scalability, automation bias and explainability. So I mentioned before that there's a risk of promoting issues that genomics research already faces, that over-sampling of certain populations. So if we take what genomics can teach us based on mostly European ancestry data, we end up imposing assumptions on populations across the globe. The role of AI here is in scaling the impact of those assumptions, taking bias algorithmic models, and applying them to diverse communities within and beyond the UK, risks not identifying certain conditions, missing patterns, potentially informing poor medical practices, if we take these bias data samples and ultimately algorithms. So the issue here about scalability is that artificial intelligence promotes the limitations of genomics research.
The second issues I mentioned on automation bias is about individuals, people potentially valuing the output of computational systems because they're mathematical and therefore might seem objective. And the challenge here is very real, if we have a clinician who is diagnosing someone and the clinician says no, there's no clear evidence for there being cancer. Following all the metrics that this clinician has learnt over the years of their work, and they're faced with an AI tool that says that this agrees that says actually there is a case for there being cancer or whatever the other option is. So the automation bias there, if it were to kick in, would be for the clinician to raise their hands, give up and say, "Well, the machine says that there is..." or "there isn't cancer, so we'll just go with what it says." The other option of is for the clinician to actually challenge what the AI tool says. And the crucial difference here is that the rationale of the clinician can be described, it can be outlined, explained. And that's the third issue, that's the issue of explainability.
So modern AI tools tend to use an enormous data set and neural networks or other machine learning technologies where outputs are produced with little or no explanation. The clinician can explain why they decided on one diagnosis or another, the AI tool cannot. Ideally, this is the really tricky bit, hospitals, Genomics England and others, would have the government structures in place to handle these discrepancies in outputs from clinicians who can explain what they have to say, and AI tools which are mathematically very sophisticated, they sound pretty cool, it's a challenge.
Natalie Banner: It absolutely is a challenge, and very helpful to talk through some of those broader ethical issues and questions. Because they are, they're questions to what I understand, you know, the law and regulation hasn't caught up yet with these very, very rapidly advancing tools and technologies. And actually, if we are working at the frontier of some of these, then these ethical questions are precisely the ones that we need to work how to navigate through. Not necessarily because of a regulatory structure, but just through bringing different voices, different perspectives to the table, trying to anticipate consequences, and thinking through where some of those questions, for example, as you raised on explainability, what could we do? Where could we address some of those challenges?
Francisco Azuaje: Yes. The issue of transparency is crucial, not only to ensure that we have useful tools, but to ensure that we improve privacy, that we respect the uses of these technologies. At the same time regardless of the techniques that we use to make systems more explainable or interpretable, the idea behind transparency also means, let's ensure that if we say that something works well, indeed, we are providing evidence that that something is working well. That means that we ensure that first of all we have reliable and robust systems, and that by doing that we are also bringing actual benefits to patients and society. So I think that's a more fundamental question than discussing which techniques can make this model or that model more explainable, or the actual practices for making something more transparent. So in general this transparency is there because we want to ensure that we deploy ethical, robust and fair systems. And that this starts by enhancing the quality and transparency of the development of the tool, but also the evaluation of those tools before their deployment, and even after these systems have been deployed to a research environment or to a clinical setting.
Ismael Kherroubi García: It sounds like there's a need for continuous monitoring, right. Throughout the life cycle of developing an AI tool, but also once implemented how we get feedback, so that the tool can be improved, but also future and other tools can be improved.
Natalie Banner: Thank you so much. So we have had a real whistle stop tour through the world of AI and genomics. We've highlighted some real potential advances in exciting areas. We've cautioned about some of the risks and questions about how to tackle some of the ethical complexities that are emerging. So just to wrap us up, Francisco, can I turn to you first, could you tell us what you see as being the biggest or the most significant impacts in the world of AI and genomics in the next, say, three to give years?
Francisco Azuaje: In the next three to five years, we should expect significant advances in genomics, AI genomics, beyond the focus individual genes or markers or actually the idea of gene panels. So we should expect that the full patient genomic analysis will become more common to provide a more comprehensive view of genetic influences on health, and also the combination of genomic data with other types of health information will offer deeper insights for supporting more accurate, faster medical decision-making.
The challenge lies in connecting this data to clinical decisions moving beyond diagnosis to actually recommend personalised treatment options. Matching patients with relevant clinical trials based on their genomic and other types of clinical information will also become more effective, more efficient. However, concerns about reliability, safety of these applications remain, and I think that in the next few years we will see an acceleration in the development of tools and applications. But also an improvement in the way we evaluate these tools before they are deployed to a real-world environment. So this will be crucial in the next few years, and despite all these challenges, there is reason to be very optimistic about the future of AI in genomics and medicine for the benefit of patients.
Natalie Banner: Thank you, Francisco. And Ismael, a last word to you, what's your key takeaway for those developing AI tools for use in genomics?
Ismael Kherroubi García: For me the biggest challenge is that there must be multidisciplinary approaches, so those developing these tools need to speak with one another and be exposed to patients. So, on the one hand, AI tools for medical applications must involve multidisciplinary collaborations, critically including the voices of clinicians, and that point was raised by Francisco. And the COVID-19 pandemic, to work with something... work for an example, already showed us the value of behavioural and other social sciences in understanding the impacts of public health policies. So genomics and general genomics research must consider multidisciplinarity in a similar way and bring different disciplines together.
On the other hand, genomics data remain intricately linked with individuals. Research participants and patients must be kept abreast of developments in the complex space that is this interaction of AI and genomics to avoid the trust issues mentioned earlier on. Ultimately, those developing AI tools for use in genomics must follow inclusive practices.
Natalie Banner: We'll wrap up there. Thank you to our guests, Ismael Kherroubi García and Francisco Azuaje for joining me today as we discussed the role of AI in genomics and healthcare, and the importance of having open, informative conversations about both the promises and the challenges in this exciting space. If you'd like to her more like this, please subscribe to the G Word on your favourite podcast app. Thank you for listening, I've been your host, Natalie Banner.
No comments yet. Be the first to say something!